Why Trust Matters in AI
Artificial Intelligence (AI) is transforming industries, delivering unprecedented efficiency, insights, and innovation. However, as AI systems become increasingly complex, they often operate as “black boxes”—producing outputs without clear explanations. This lack of transparency creates a significant trust barrier, especially for high-stakes applications like healthcare, finance, and recruitment. Without trust, AI adoption slows, limiting its transformative potential.
Despite the rush to implement AI solutions, trust remains a critical challenge. According to McKinsey’s 2024 research, only 17% of organizations are actively addressing explainability, despite 40% identifying it as a crucial risk. To bridge this trust gap, organizations are turning to Explainable AI (XAI), which makes AI systems more transparent, fostering confidence among users, stakeholders, and regulators.
The Growing Demand for Explainable AI
In today’s rapidly evolving technological landscape, AI has become a cornerstone of business transformation. However, a striking statistic from McKinsey reveals that 91% of organizations doubt their readiness to implement AI safely and responsibly. The solution? Explainable AI (XAI)—the bridge between powerful AI capabilities and human trust.
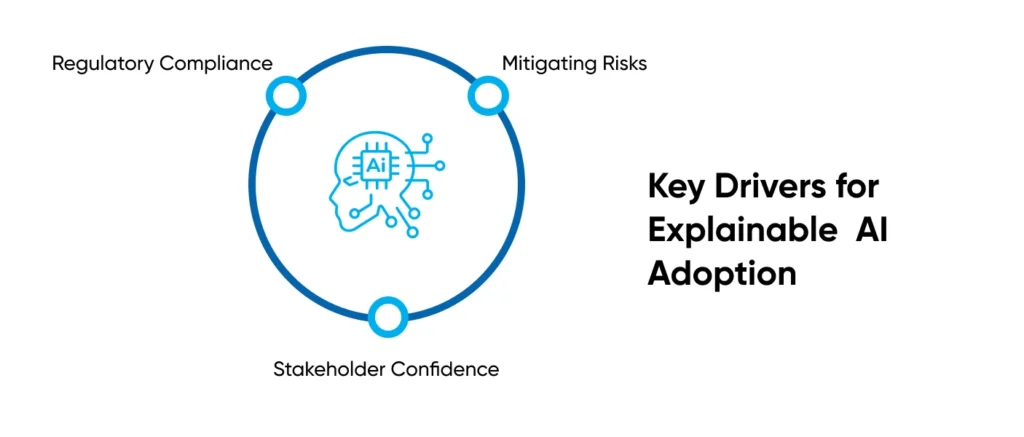
- Regulatory Compliance:
Global regulations, like the EU AI Act, mandate transparency in AI models used in sensitive areas such as hiring and credit scoring. - Mitigating Operational Risks:
Transparent models help businesses reduce errors, bias, and inaccuracies in AI-driven decisions. - Boosting Stakeholder Confidence:
Users and executives are more likely to adopt AI when they understand how it works.
What is Explainable AI (XAI)?
Explainable AI (XAI) refers to a set of tools and techniques that help users understand the reasoning behind an AI model’s outputs. Unlike traditional AI, which often lacks transparency, XAI sheds light on complex algorithms by offering insights into their decision-making processes.
Benefits of XAI
- Improved Trust: Users are more likely to trust AI when its decisions are explainable.
- Better Debugging: Developers can identify and fix issues faster with clear insights into AI behavior.
- Enhanced Fairness: Explainability helps organizations detect and reduce bias in AI models.
Why XAI is Critical for Business Success
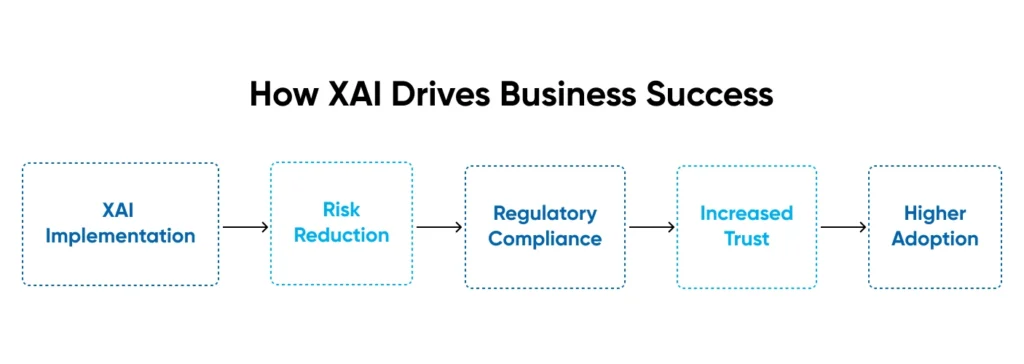
1. Operational Risk Mitigation
AI models, especially in financial services, often make critical decisions like fraud detection or loan approvals. Without XAI, organizations face risks like false positives, which can harm customer relationships and result in losses.
2. Regulatory Compliance
Many industries, including healthcare and human resources, are governed by strict regulations. XAI ensures models comply with these rules by providing clear explanations for decision-making.
3. Stakeholder Confidence and Adoption
When executives, end-users, and regulators can understand AI’s logic, they are more likely to trust and adopt AI solutions.
Key Techniques and Tools for Explainable AI
1. Global vs. Local Explainability
- Global Explainability: Offers insights into the overall behavior of an AI model. Ideal for understanding broad patterns in decision-making.
Example: A bank using AI for loan approvals can see which factors (income, credit score) influence approvals across all customers. - Local Explainability: Focuses on explaining specific predictions.
Example: In healthcare, a doctor can understand why an AI system predicted a particular diagnosis for an individual patient.
2. Popular Tools for XAI
- LIME (Local Interpretable Model-agnostic Explanations): Explains individual predictions by approximating a complex model with an interpretable one.
- SHAP (Shapley Additive Explanations): Provides consistent, accurate explanations by assigning importance scores to each feature.
- Google’s What-If Tool: Allows users to test AI models with hypothetical scenarios to see how they respond.
- IBM’s AI Explainability 360: An open-source toolkit that helps in understanding and explaining AI models.
Implementing Explainability in AI Development
To embed explainability in AI solutions, organizations should take the following steps:
- Build Cross-Functional Teams
Include data scientists, engineers, compliance officers, and UX designers to ensure a well-rounded approach. - Define Clear Objectives
Identify which stakeholders need explanations and what level of detail is required. - Select or Build the Right Tools
Adopt tools like LIME, SHAP, or IBM’s toolkit that best fit the organization’s AI models and use cases. - Monitor and Iterate
Continuously gather feedback from stakeholders and refine explainability methods.
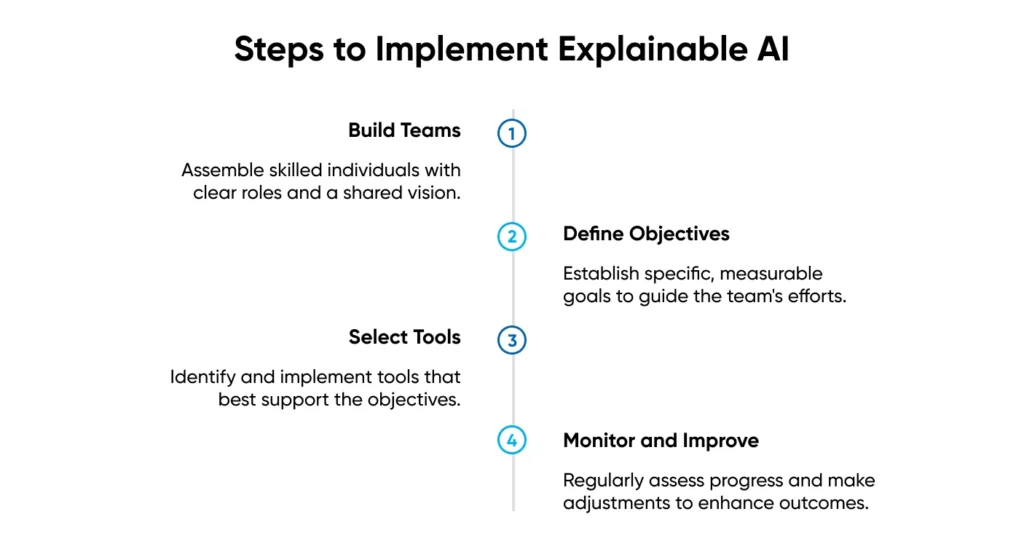
Conclusion: Trust, Transparency, and Growth
The future of AI hinges on trust. Explainable AI is not just a technical requirement—it’s a strategic imperative. By embedding explainability into AI systems, businesses can ensure compliance, improve user adoption, and foster confidence among stakeholders.
Companies that prioritize XAI will gain a competitive edge, driving innovation while maintaining transparency and accountability. Trust is the bridge between transformative AI technologies and their successful adoption, and XAI is the key to building that bridge.