Why Traditional Forecast Fails?
For decades, retailers have relied on forecasting methods rooted in historical sales data and, often, gut instinct. While these traditional approaches served their purpose in a simpler era, today’s retail landscape is vastly different. We face rapidly changing consumer trends, omnichannel complexities, shorter product lifecycles, and unprecedented supply chain volatility.
Traditional retail demand forecasting often struggles because it:
- Operates at an Aggregate Level: Forecasting for entire categories or stores misses the nuances between individual products (SKUs) or specific locations.
- Relies Heavily on Past Data: It struggles to predict the impact of new trends, sudden market shifts, competitor actions, or promotional activities accurately.
- Is Slow and Reactive: Manual adjustments and periodic updates can’t keep pace with real-time market dynamics, leading to costly delays.
The result? The all-too-familiar pains of overstocking (tying up capital, increasing holding costs, risking obsolescence) and stockouts (leading to lost sales, frustrated customers, and damaged brand loyalty). In this environment, guesswork isn’t just inefficient; it’s detrimental to the bottom line.
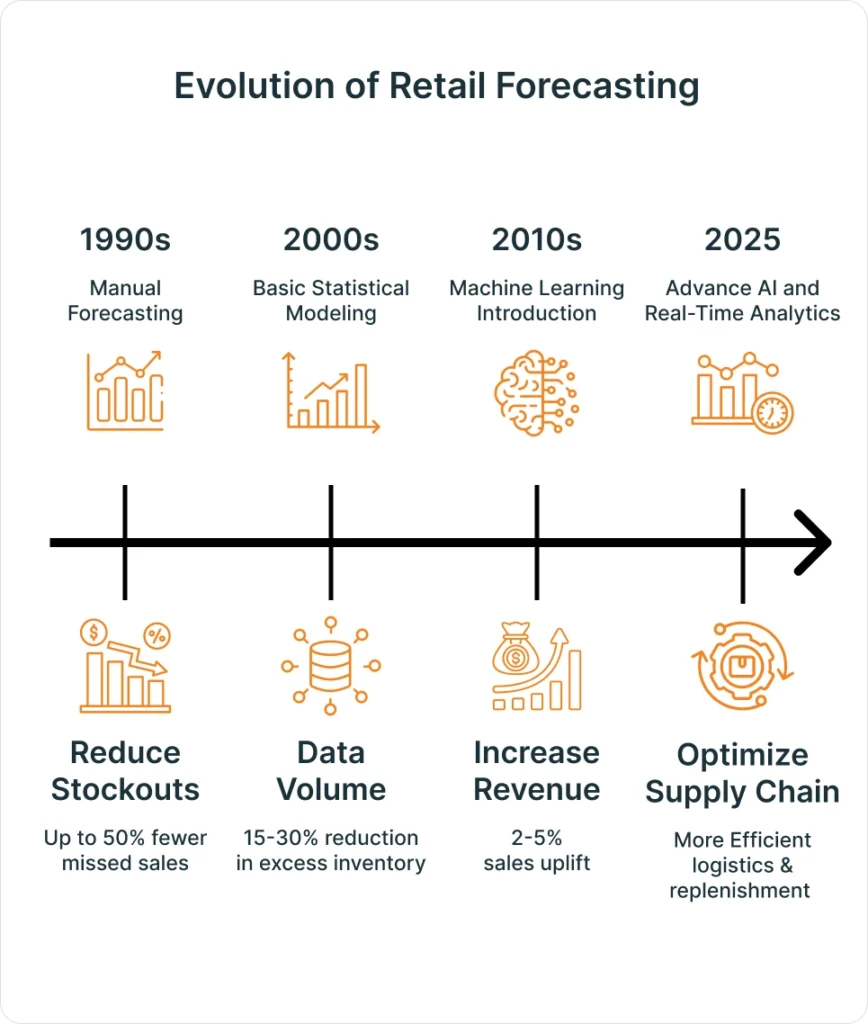
Fine-Grained Inventory Prediction
Enter Artificial Intelligence (AI) and Machine Learning (ML). AI isn’t just an incremental improvement; it represents a paradigm shift in AI inventory management. By leveraging sophisticated algorithms, AI can analyze vast and diverse datasets far beyond simple historical sales figures. This includes:
- Point-of-sale (POS) data
- Website traffic and online behaviour
- Social media trends and sentiment
- Competitor pricing and promotions
- Weather patterns
- Economic indicators
- Supply chain disruptions
- Marketing campaign data
The real game-changer, however, is AI’s ability to perform fine-grained inventory prediction. Instead of forecasting demand for “blue sweaters” across a region, AI can predict demand for a specific blue sweater SKU, in a specific size, at a specific store location or distribution center, sometimes even predicting hourly fluctuations.
What Makes Fine-Grained Forecasting So Powerful?
- SKU-Level Accuracy: Understand demand for each unique product, minimizing waste and maximizing availability where it counts.
- Location Specificity: Account for variations in demand driven by local events, demographics, or store-specific promotions.
- Real-Time Adaptability: AI models constantly learn and refine predictions as new data flows in, reacting dynamically to changing conditions.
This level of granularity allows retailers to move from reactive stock adjustments to proactive, data-driven inventory optimization.
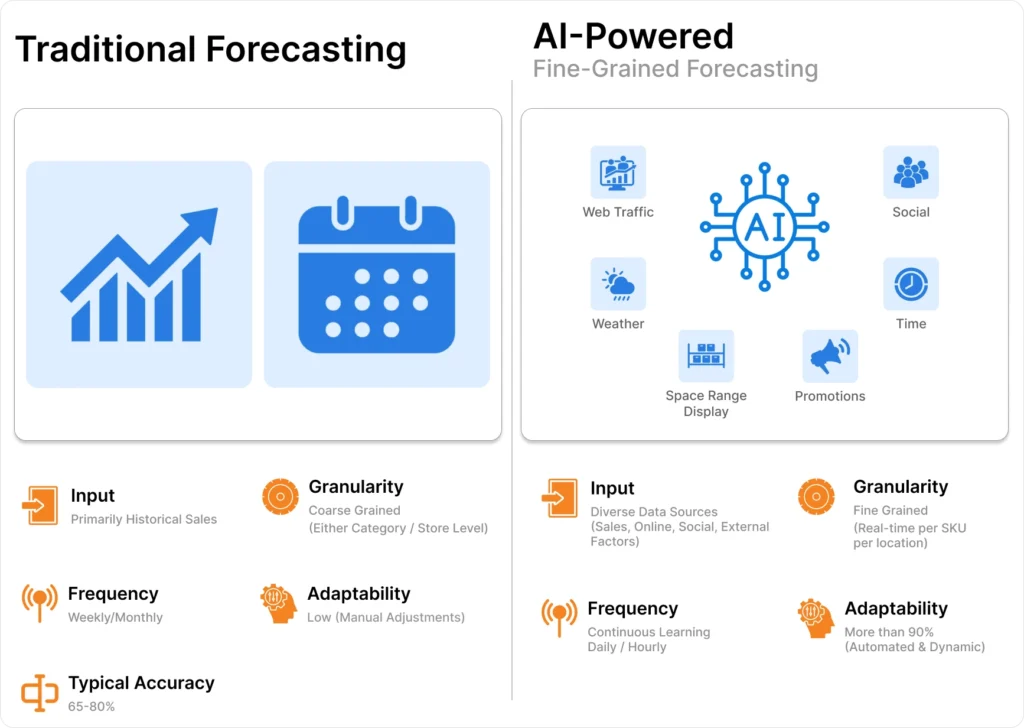
The Role of Advanced Data Platforms: Enabling Scale and Speed with Databricks
Achieving true fine-grained, real-time retail demand forecasting requires a robust platform capable of handling massive datasets and complex computations. This is where platforms like Databricks become essential.
Databricks provides a unified environment that excels at:
- Ingesting and Processing Diverse Data: Seamlessly integrating structured data (like sales transactions) with unstructured data (like social media comments or weather reports) at scale.
- Scalable Machine Learning: Offering powerful tools and infrastructure (like managed MLflow and optimized Spark clusters) to build, train, and deploy sophisticated AI/ML forecasting models efficiently.
- Collaboration and Iteration: Enabling data scientists, engineers, and business analysts to work together on a single platform, speeding up the development and refinement of forecasting models.
- Real-Time Capabilities: Supporting streaming data processing and real-time model inference, crucial for keeping forecasts up-to-date with the latest market signals.
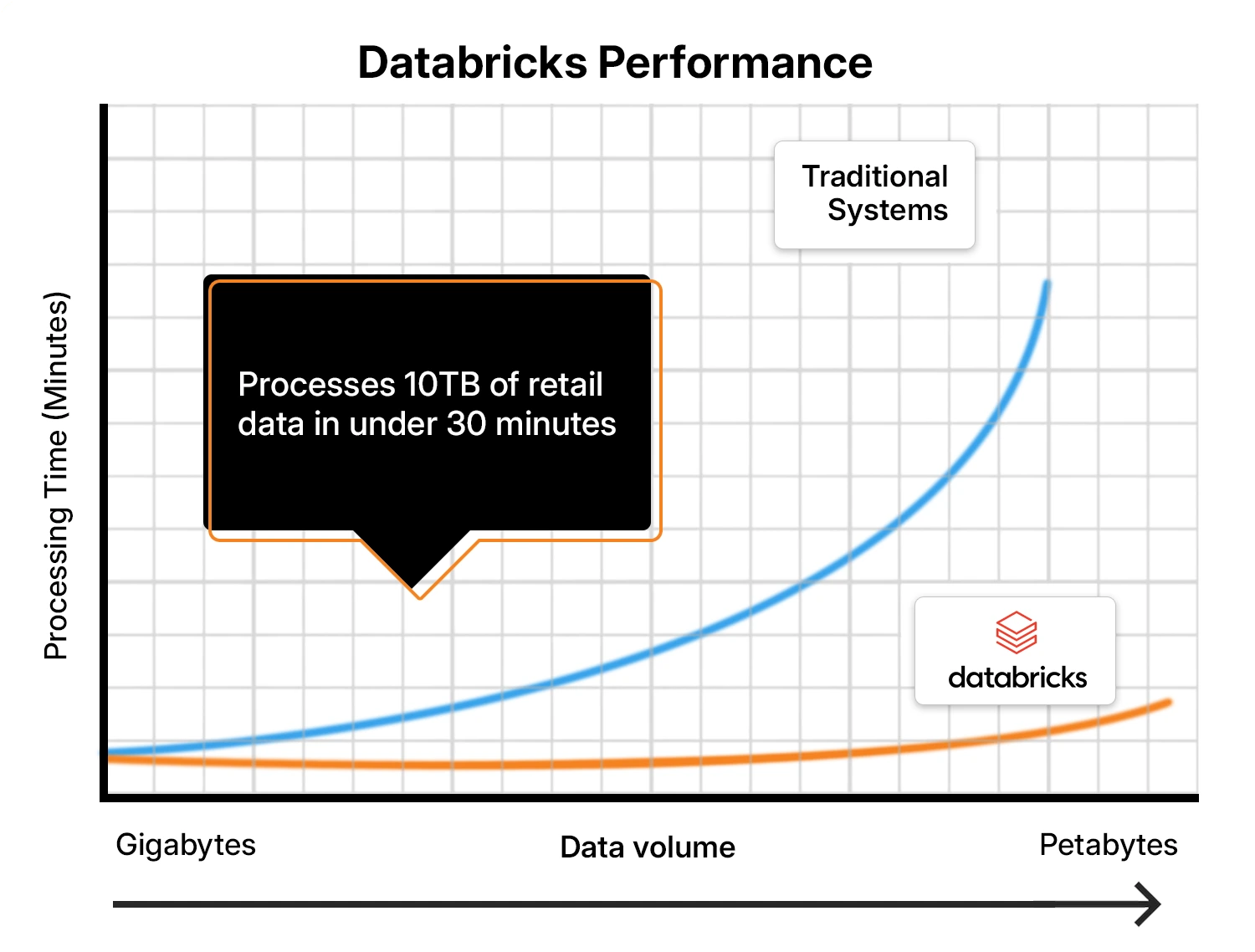
By leveraging platforms like Databricks, retailers can overcome the technical hurdles associated with implementing advanced AI inventory management, unlocking the full potential of fine-grained inventory prediction.
What AI-Driven Forecast Delivers ?
Adopting AI-powered, fine-grained forecasting isn’t just about better predictions; it’s about driving concrete business results:
- Reduced Stockouts: Increase product availability and capture previously lost sales (potentially reducing stockouts by 30-50%).
- Minimized Overstocking: Lower holding costs, reduce markdowns, and decrease waste (potentially cutting excess inventory by 15-30%).
- Improved Margins: Optimize pricing and promotions based on accurate demand signals.
- Increased Sales: Ensure the right products are in the right place at the right time, boosting overall revenue (often seeing a 2-5% lift).
- Enhanced Customer Satisfaction: Provide a more reliable shopping experience, building loyalty.
- Optimized Supply Chain: Improve logistics planning, warehouse utilization, and replenishment efficiency.
- Better Staff Allocation: Align staffing levels with predicted store traffic and demand peaks.
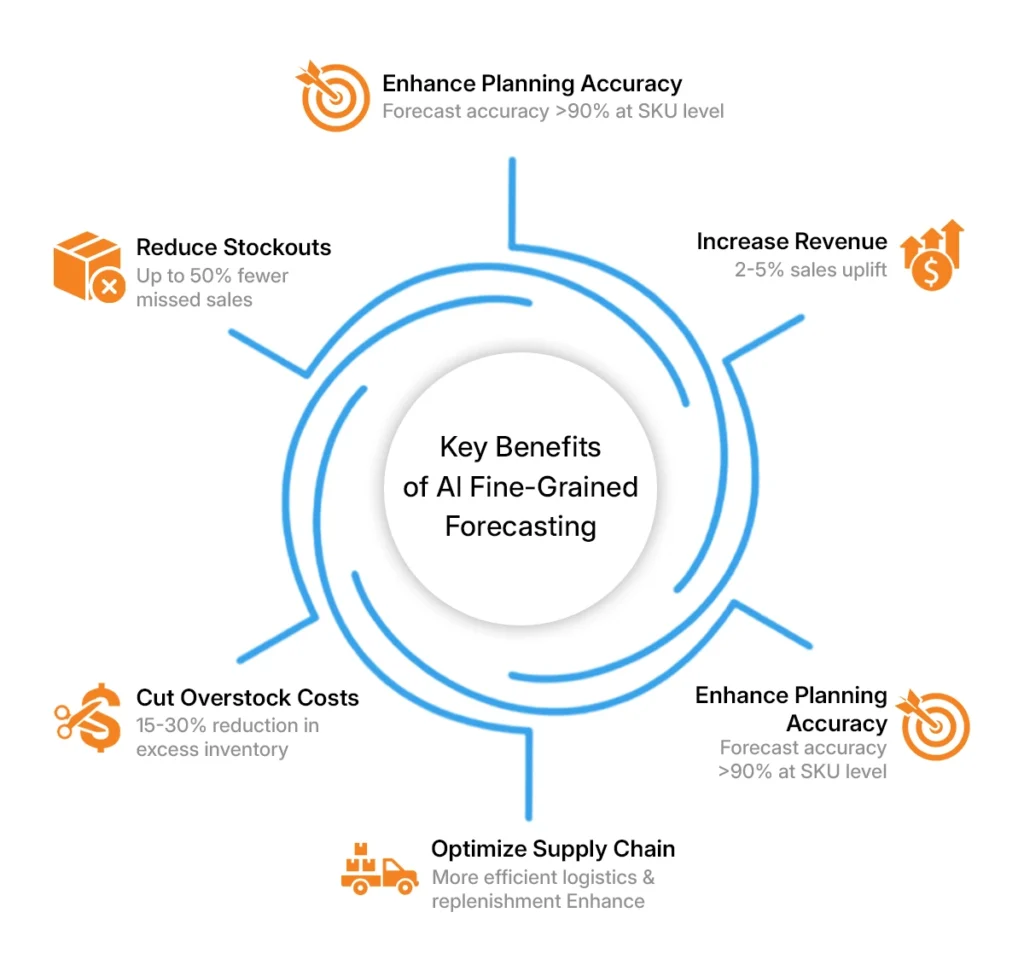
Getting Started: Embracing the Future of Retail Inventory
Transitioning to AI-driven forecasting requires a strategic approach:
- Data Foundation: Ensure access to clean, reliable, and diverse data sources. Data quality is paramount.
- Technology Partnership: Select the right AI platform and tools (like Databricks) that fit your scale and technical capabilities.
- Start Focused: Begin with a pilot project on a specific product category or region to demonstrate value and refine processes.
- Cross-Functional Collaboration: Involve teams from merchandising, supply chain, IT, and marketing from the outset.
- Change Management: Train teams on how to interpret and trust AI-driven insights, shifting from intuition-based decisions to data-driven strategies.
Conclusion: The Inevitable Shift Towards Intelligent Retail
The era of broad-stroke, backward-looking retail demand forecasting is fading. AI-powered, fine-grained inventory prediction offers unprecedented accuracy, agility, and efficiency. Retailers embracing these technologies, supported by powerful platforms like Databricks, are not just optimizing their inventory; they are building a more resilient, responsive, and profitable business poised for future success. Adapting to this intelligent approach is no longer optional – it’s essential for survival and growth in the competitive retail landscape.
Frequently Asked Questions
Are ready to level up your retail business? Contact us to learn more about implementation strategies and success stories.